Applications of NLP in healthcare: how AI is transforming the industry
Natural Language Processing has been a recent game-changer in healthcare. Let’s now explore both its applications and challenges a bit further.
31 March, 2023Natural Language Processing has been a recent game-changer in healthcare, allowing healthcare professionals to analyze vast amounts of data, including patient data, clinical research, and medical records, and then transforming it into actionable insights.
This technology is also the driving force behind building an AI assistant, which can help automate many healthcare tasks, from clinical documentation to automated medical diagnosis.
Healthcare AI companies now offer custom AI solutions that can analyze clinical text, improve clinical decision support, and even provide patient care through healthcare chatbot applications.
However, as with any new technology, there are challenges to be faced in implementing NLP in healthcare, including data privacy and the need for skilled professionals to interpret the data. Let’s now explore both the applications and challenges a bit further.
Uses of NLP in healthcare
NLP has a wide range of applications in healthcare. Here’s an overview of the most popular ones.

Clinical documentation
Clinical documentation is a crucial aspect of healthcare, but it can be time-consuming and error-prone when done manually. NLP technology is being used to automate this process, enabling healthcare professionals to extract relevant information from patient records and turn it into structured data, improving the accuracy and speed of clinical decision-making.
NLP algorithms can also assist with coding diagnoses and procedures, ensuring compliance with coding standards and reducing the risk of errors. They can also help identify potential safety concerns and alert healthcare providers to potential problems.
Improving clinical decision support
This technology also enhances clinical decision support by extracting relevant information from patient records and providing insights that can assist healthcare professionals in making informed decisions. By analyzing large amounts of unstructured data, NLP algorithms can identify patterns and relationships that may not be immediately apparent to humans.
This can lead to more accurate diagnoses, earlier detection of potential health risks, and more personalized treatment plans. Additionally, NLP can help identify gaps in care and suggest evidence-based interventions, leading to better patient outcomes.
Automated medical diagnosis
Next application is the ability to automate medical diagnosis, enabling healthcare professionals to quickly and accurately diagnose patients. The algorithms can analyze large amounts of unstructured data, such as medical records and clinical notes, and identify patterns and relationships that can aid in diagnosis.
Along with faster diagnoses, earlier detection of potential health risks, and more personalized treatment plans, NLP can also help identify rare diseases that may be difficult to diagnose and can suggest relevant tests and interventions.
Automating medical records
NLP technology also has the potential to automate medical records, giving healthcare providers the means to easily handle large amounts of unstructured data. By extracting information from clinical notes, NLP converts it into structured data, making it easier to manage and analyze.
This automation can also reduce the time spent on record-keeping, allowing one to focus more on patient care. Plus, automating medical records can improve data accuracy, reduce the risk of errors, and improve compliance with regulatory requirements.
Patient care
Another use of NLP technology involves improving patient care by providing healthcare professionals with insights to inform personalized treatment plans. By analyzing patient data, NLP algorithms can identify patterns and relationships that may not be immediately apparent, leading to more accurate diagnoses and treatment plans.
NLP can also aid in identifying potential health risks and providing targeted interventions to prevent adverse outcomes. It can also be used to develop healthcare chatbot applications that provide patients with personalized health information, answer common questions, and triage symptoms.
Clinical case study
In clinical case research, NLP is used to analyze and extract valuable insights from vast amounts of unstructured medical data such as clinical notes, electronic health records, and patient-reported outcomes. NLP tools can identify key medical concepts and extract relevant information such as symptoms, diagnoses, treatments, and outcomes.
These insights can then improve patient care, clinical decision-making, and medical research. NLP can also help clinicians identify patients at risk of developing certain conditions or predict their outcomes, allowing for more personalized and effective treatment.
Clinical text analysis
NLP is now an essential tool for clinical text analysis, which involves analyzing unstructured clinical text data like electronic health records, clinical notes, and radiology reports. It does so by extracting valuable information from these texts, such as patient demographics, diagnoses, medications, and treatment plans.
NLP can also help identify key phrases and patterns in the data, which can be used to inform clinical decision-making, identify potential adverse events, and monitor patient outcomes. Additionally, it assists in improving the accuracy and efficiency of clinical documentation.
Healthcare chatbot applications
Finally, this technology is being utilized to develop healthcare chatbot applications that can provide patients with personalized health information, answer common questions, and triage symptoms. It analyzes patient data and understands natural language queries to then provide patients with accurate and timely responses to their health-related inquiries.
Chatbots can also assist with scheduling appointments, refilling prescriptions, and providing medication reminders, improving patient engagement and adherence to treatment plans.
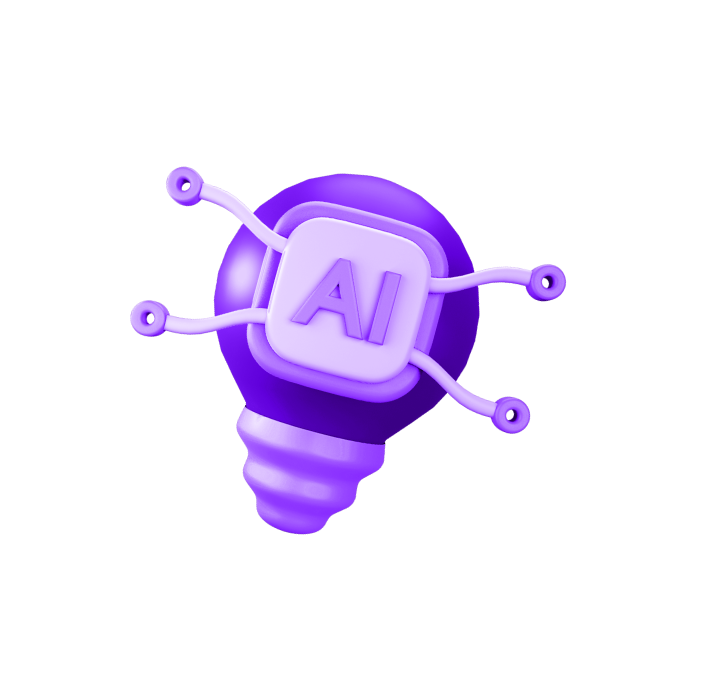
What is Natural Language Processing (NLP)?
Here’s a brief look into the origins of NLP and how it essentially works:
- Natural Language Processing focuses on enabling machines to understand, interpret, and generate human language. The roots of NLP can be traced back to the early 1950s when researchers began exploring the possibility of creating machines that could understand human language. Over the decades, advances in machine learning, linguistics, and computer science have enabled the development of more sophisticated NLP algorithms and tools.
- NLP consists of several tasks: text classification, sentiment analysis, language translation, and speech recognition. These tasks are carried out by NLP tools, including part-of-speech taggers, named entity recognizers, and parsers. The approaches can be divided into rule-based and statistical methods. Rule-based methods rely on manually crafted rules to process language, while statistical methods use machine learning algorithms to automatically learn patterns from data.
- It works by breaking down language into its constituent parts and analyzing them. This involves identifying the parts of speech, detecting named entities, parsing sentence structures, and extracting meaning from text. NLP algorithms are trained on large amounts of annotated data, which enables them to learn how to process language and identify patterns.
Examples of NLP in healthcare
Some of the newest and most relevant examples of NLP in healthcare include:
- COVID-19 diagnosis - NLP algorithms are used to develop automated tools for diagnosing COVID-19 based on chest X-rays and CT scans.
- Clinical document analysis - NLP analyzes electronic health records (EHRs) and extracts valuable insights, such as trends and patterns, to inform treatment plans.
- Medication adherence - NLP-powered chatbots are being used to improve medication adherence by providing patients with personalized reminders and education.
- Clinical trials, such as extracting relevant information from clinical trial protocols and identifying potential eligibility criteria for participants.
- Telemedicine - NLP enables real-time voice-to-text transcription and facilitates more accurate and efficient communication between patients and healthcare professionals.
Challenges of NLP in healthcare
While NLP has great potential to revolutionize the healthcare industry, several challenges still need to be addressed:
- Data quality
Healthcare data is often messy, incomplete, and difficult to process, so the fact that NLP algorithms rely on large amounts of high-quality data to learn patterns and make accurate predictions makes ensuring data quality critical.
- Privacy and security
Healthcare data is highly sensitive and subject to strict privacy and security regulations. NLP systems must be designed to protect patient privacy and maintain data security, which can be challenging given the complexity of healthcare data and the potential for human error.
- Bias
NLP algorithms can reflect the biases present in the data used to train them. In healthcare, this can lead to inaccurate diagnoses or treatments, particularly for underrepresented or marginalized groups. The algorithms should be created free from bias and reflect the diversity of patient populations.
- Integration with existing systems
Integrating NLP systems with existing healthcare IT infrastructure can be challenging, particularly given the diversity of systems and data formats in use. NLP solutions must be designed to integrate seamlessly with existing systems and workflows to be effective.
- Interpretability
NLP algorithms can be complex and difficult to interpret, which can limit their usefulness in clinical decision-making. NLP models that are transparent and interpretable are critical for ensuring their acceptance and adoption by healthcare professionals.
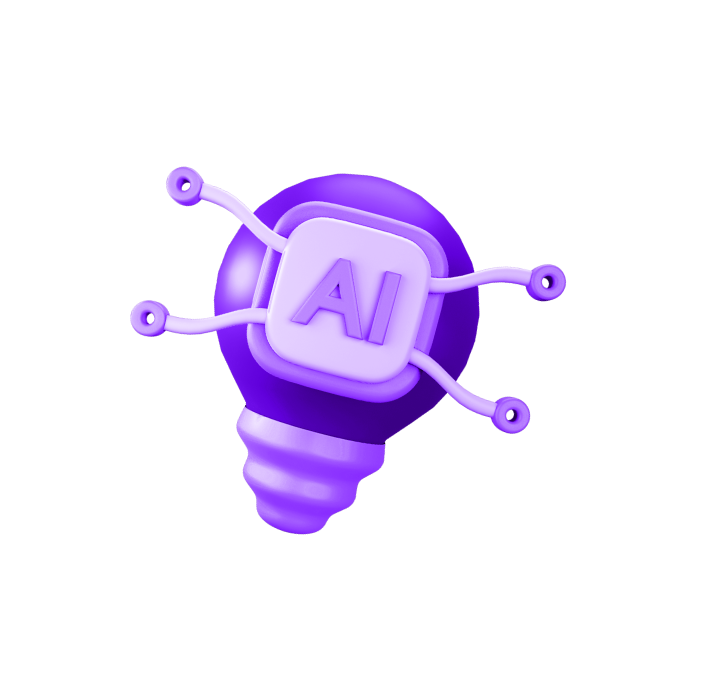
NLP is here to stay in healthcare
In conclusion, NLP thoroughly shakes up healthcare by enabling new and innovative approaches to diagnosis, treatment, and patient care. While some challenges remain to be addressed, the benefits of NLP in healthcare are pretty clear.
From improving clinical decision-making to automating medical records and enhancing patient care, NLP-powered tools and technologies are finally breaking the mold in healthcare and its old ways.
As the industry continues to embrace AI and machine learning, NLP is poised to become an even more important tool for improving patient outcomes and advancing medical research.
So, in short, NLP is here to stay in healthcare and will continue to shape the future of medicine.