AI in healthcare: discovering the benefits and challenges
From improving patient care to revolutionizing disease treatment, we will uncover how AI is reshaping the future of healthcare.
26 May, 2023Just like AI tools for business, the medical universe also benefits from AI's integration. The amalgamation of artificial intelligence and healthcare is now continuously bringing new gems. We now see algorithms playing doctor and data becoming the ultimate healer. Pretty exciting, not gonna lie!
Brace yourself for a journey through the benefits and challenges that AI brings to the medical landscape. From improving patient care to revolutionizing disease treatment and prevention, we will uncover how AI is reshaping the future of healthcare and donning its superhero cape to battle ailments. And stay tuned for examples of AI in finance in our next article.
Benefits of AI in healthcare
Every reputable AI agency is now on a mission to uncover the best uses of this technology in healthcare. Here are the main four that are unbeatable at the moment.

Streamlining diagnoses
In AI-powered healthcare, streamlining diagnoses emerges as a major benefit, revolutionizing the diagnostic process.
A new era of efficiency unfolds through the seamless collaboration between advanced AI algorithms and medical data, where the former sifts through mountains of information and identifies patterns and anomalies that elude human eyes.
This, in turn, empowers physicians to make more accurate and timely diagnoses of complex diseases and ensures that patients receive tailored treatment plans personalized to their unique needs.
Automating administrative tasks
The automation of administrative tasks is also a game-changer when it comes to the efficiency and productivity of medical workflows.
With the aid of AI, the once arduous and time-consuming administrative processes transform into a streamlined symphony. AI algorithms deftly navigate through tons of paperwork, extracting and organizing essential information with remarkable accuracy.
As a result, tedious tasks like scheduling appointments, managing patient records, and processing insurance claims become a breeze. This automation allows healthcare professionals to focus more on patient care.
Improving patient care
Through continuous monitoring and analysis of patient data, AI alerts healthcare professionals to potential risks and deviations. This proactive approach enables timely interventions, ensuring patient safety and well-being.
AI also empowers clinicians with evidence-based insights, enabling more informed decision-making and personalized treatment plans tailored to each patient's unique needs.
And don’t forget AI-driven chatbots and virtual assistants that provide round-the-clock support, offering patients access to immediate medical information and guidance.
Beyond traditional healthcare settings, AI-driven tools are transforming specialized practices, including Applied Behavior Analysis (ABA) therapy. In ABA practices, AI enhances treatment planning, progress tracking, and data analysis, ensuring more effective therapy for individuals with autism and other developmental disorders. Platforms like Theralytics ABA practice management solution streamline administrative tasks, automate data collection, and provide actionable insights, allowing clinicians to focus more on patient care and improve therapy outcomes.
Artificial Intelligence in disease treatment and prevention
In disease treatment and prevention, AI is now catalyzing once unimaginable advancements. We've already mentioned its help in identifying patterns, trends, and correlations that lead to more accurate diagnoses, optimal treatment plans, and predicting disease progression.
Early detection and risk assessment give a better opportunity to enable proactive interventions and lifestyle modifications. AI-driven algorithms also allow the discovery of novel therapies and pharmaceutical compounds, accelerating the development of groundbreaking treatments.
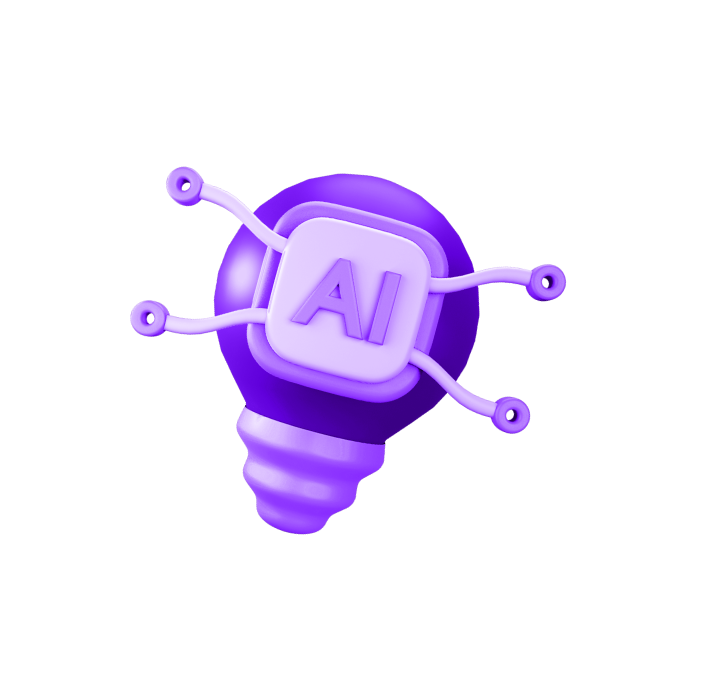
Machine learning with healthcare data
Without beating around the bush, here's why Machine learning is a cornerstone of AI in healthcare:
- Machine learning thrives on large volumes of diverse healthcare data. With the exponential growth of electronic health records (EHRs), genomic data, medical imaging, wearable devices, and more, machine learning algorithms can extract valuable insights from these vast datasets.
- By analyzing historical patient data, lifestyle factors, genetic information, and environmental factors, ML algorithms can identify patterns and indicators that aid in predicting the likelihood of developing certain conditions. Early intervention becomes possible, improving patient outcomes and reducing healthcare costs.
- Machine learning also enables the customization of treatment plans based on individual patient characteristics. It can recommend tailored interventions, medication regimens, and therapies based on genetic markers, patient demographics, medical history, and treatment responses.
- Through deep learning techniques, ML algorithms can analyze radiology images, pathology slides, and other medical images, aiding in detecting abnormalities, early-stage cancers, and other critical conditions.
- And finally, ML can integrate with wearable devices and remote monitoring systems to offer the potential for real-time health tracking. It can continuously collect and analyze patient data, such as heart rate, blood pressure, glucose levels, and activity patterns, helping to identify anomalies and trigger alerts for healthcare providers.
Challenges for Artificial Intelligence in healthcare
Sadly, some limitations are expected with any kind of technology.

Algorithmic limitations
Many AI algorithms, such as deep learning neural networks, operate as black boxes, making it challenging to understand and interpret their decision-making processes. Lack of transparency raises concerns about trust, accountability, and the ability to explain the reasoning behind AI-generated predictions or recommendations.
They may also struggle with generalizing findings from one population or healthcare setting to another. Bias can inadvertently arise if algorithms are trained on biased or incomplete data, leading to disparities in diagnostic accuracy or treatment recommendations across different demographic groups.
The same with the quality, completeness, and representativeness of healthcare data. Insufficient or biased data can hamper algorithm training, leading to suboptimal performance or even erroneous results.
Potential approaches to address these limitations include developing algorithms that provide transparent explanations for their decisions, improving data quality and diversity, and establishing rigorous evaluation and validation frameworks.
Regulatory barriers
AI algorithms rely on vast amounts of patient data, including sensitive health information. Compliance with privacy regulations, such as HIPAA or GDPR, poses serious challenges, and ensuring data security, obtaining patient consent, and anonymizing or de-identifying data while maintaining its utility for AI applications can be quite complex.
Remember that AI systems intended for use in healthcare, especially those involved in diagnostics, treatment planning, or medical decision-making, may also need rigorous regulatory approval processes, similar to other medical devices or drugs. Ensuring the safety, effectiveness, and reliability of AI algorithms becomes crucial, necessitating regulatory oversight and proper validation procedures.
One way to fix this limitation is developing and adapting regulations to account for the unique characteristics and challenges of AI in healthcare while ensuring patient safety, data privacy, and ethical standards. Another helpful technique is creating regulatory sandboxes and pilot programs that enable controlled testing and evaluation of AI technologies.
Misaligned incentives
Misaligned incentives refer to the disconnect between stakeholders' goals and motivations in adopting AI in healthcare. Providers may face financial and adoption challenges, while reimbursement models may not correctly incentivize AI integration.
Data sharing barriers also hinder AI's full potential, as well as funding gaps in AI research, which may impede progress since funding mechanisms and priorities may favor more traditional research areas.
Addressing misaligned incentives requires value-based healthcare models prioritizing outcomes, collaborative partnerships among stakeholders, supportive policies and regulations, and education initiatives to increase awareness.
Data access limitations
Data access limitations are the challenges associated with accessing, sharing, and utilizing healthcare data for AI applications. They arise primarily due to privacy regulations, data governance policies, technical barriers, and fragmented data systems.
These limitations can hinder the development and implementation of AI algorithms that require extensive and diverse datasets. Restricted access to data silos, lack of interoperability among different healthcare systems, and concerns over patient privacy and data security pose significant obstacles.
Fixing this aspect may require establishing robust data governance frameworks, promoting data-sharing collaborations, ensuring data interoperability standards, and addressing privacy and security concerns.
Policy implications
Policy implications in AI healthcare involve developing ethical frameworks, regulatory guidelines, and data governance policies. Policymakers must balance innovation, patient rights, and responsible AI adoption. Key factors:
- Standardization and interoperability are crucial for seamless collaboration, while workforce training ensures healthcare professionals can effectively utilize AI.
- Patient engagement and informed consent are important for transparency and privacy protection.
- International collaboration promotes global interoperability and policy harmonization.
Real-world examples of how AI tools have affected healthcare
Here are some examples to prove that AI’s help in healthcare is not just a figment of our imagination:
- Early detection of lung cancer. AI algorithms developed to analyze medical images, such as CT scans, can accurately identify suspicious nodules or lesions.
Proof: Aidoc, an AI-powered radiology platform, or Zebra Medical Vision, which develops algorithms for the automated analysis of medical images.
- Personalized treatment recommendations. AI algorithms can suggest targeted therapies and predict the efficacy of specific treatments, leading to more precise and effective care.
Proof: an AI-enabled precision medicine platform called Tempus or Merative (previously IBM Watson Health).
- AI-powered diabetic retinopathy screening. AI tools have been deployed to analyze retinal images for the early detection of diabetic retinopathy, a leading cause of blindness.
Proof: IDx-DR, an AI diagnostic system that autonomously diagnoses patients for diabetic retinopathy and macular edema.
- Virtual assistants and chatbots. AI-powered tools can answer patient queries, schedule appointments, and offer personalized health recommendations.
Proof: Ada Health, a popular symptom assessment app, or Woebot, an AI chatbot designed to provide mental health support and guidance to users through conversational interactions.
- Predictive analytics for hospital operations. AI helps analyze hospital data, including patient flow, resource utilization, and readmission rates, to predict and optimize hospital operations.
Proof: Epic Systems helping optimize hospital operations, such as predicting patient length of stay and resource utilization, or LeanTaaS offering predictive capacity planning and patient flow optimization.
- Surgical assistance. Robotic surgical systems powered by AI algorithms can assist surgeons with real-time feedback, image analysis, and enhanced visualization, enabling minimally invasive surgeries with improved outcomes.
Proof: Intuitive, maker of the da Vinci surgical and Ion endoluminal systems, or Activ Surgical, an intraoperative surgical intelligence.
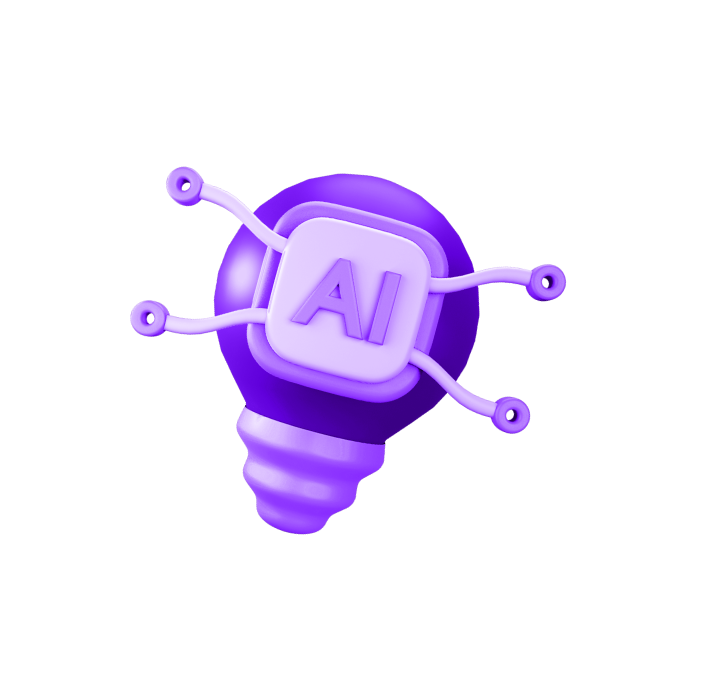
AI is here to stay in healthcare
Our conclusion is in the title. AI is definitely going to settle in. Algorithms became healgorithms, and chatbots put on stethoscopes.
And it seems like AI's ability to streamline diagnoses, automate tasks, and revolutionize patient care is just the beginning. From lung cancer detection by robot radiologists to virtual assistants offering medical advice, the future is full of exciting possibilities.
But wait, challenges like algorithmic limitations and regulatory hurdles also demand our attention. Let's align incentives, break down data barriers, and shape thoughtful policies.
You know AI isn't just a trend anymore…