Applications of NLP in business and everyday life
We explore the various applications of NLP in business and everyday life, the benefits it brings, and the future directions for NLP applications.
6 April, 2023In recent years, Natural Language Processing has emerged as a vital part of the AI development process. It has given us a way to communicate with machines in a more natural and efficient manner. One of the most exciting applications of NLP is building an AI assistant or chatbot that can understand and respond to natural language.
Speaking from experience as an AI design company, you should increasingly leverage NLP to get more sophisticated and intelligent chatbots and dialogue systems that your customers will appreciate.
We’d like to explore the various applications of NLP in business and everyday life, the benefits it brings, and the future directions for NLP applications. So let's dive into the world of NLP and discover its potential.
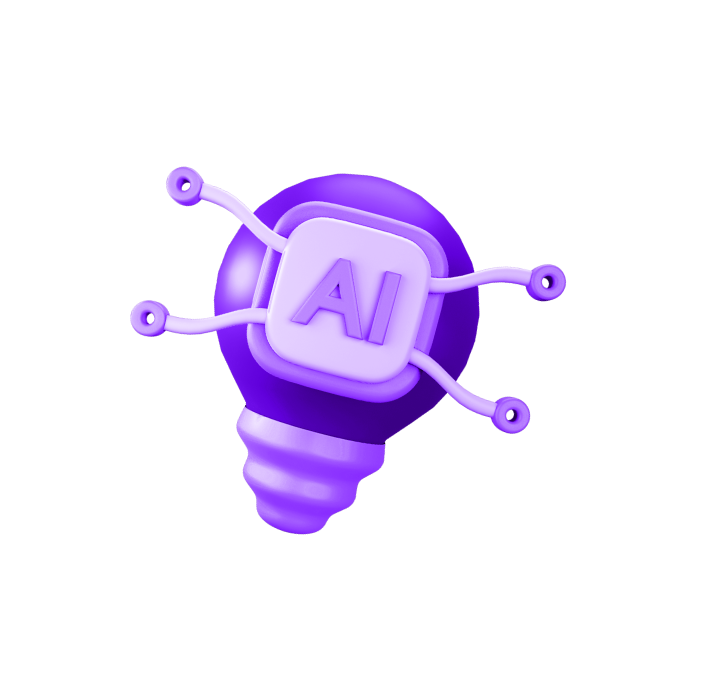
What is Natural Language Processing?
Natural Language Processing is a subfield of AI that deals with the interaction between computers and human language. NLP enables computers to understand, interpret, and generate human language.
The history of NLP dates back to the 1950s, and the technology has since then snowballed, especially with the advent of machine learning. It works by using statistical models and algorithms to identify patterns and relationships in language data.
Today, NLP is more prevalent than ever before, thanks to advancements in said machine learning and the widespread availability of data. With the rise of digital communication and the internet, vast amounts of text data are generated every day, providing a fertile ground for NLP applications.
NLP is now used in various industries, including healthcare, finance, marketing, and e-commerce. It has also found its way into everyday life, from virtual assistants like Siri and Alexa to spam filters in email clients, and there are more applications to come.
How NLP is used
Thanks to its ability to interpret human language, NLP has found various applications in both business and everyday life. Here are some of them.

Automatic summarization
Automatic summarization is a popular application of NLP that involves generating a shorter version of a text while retaining the essential information.
It is pretty convenient for processing large amounts of text data, enabling people to quickly understand the key points of an article, document, or news story, so it’s being widely used in journalism, content curation, information retrieval, etc.
Automatic summarization can be extractive, where the summary is created by selecting and combining the most important sentences or phrases from the original text, or abstractive, where a summary is created by generating new sentences that capture the essence of the original text.
Text classification and understanding
Text classification is another NLP application that involves assigning categories or labels to pieces of text to automatically classify documents, emails, or social media posts into different categories, such as spam or not spam, positive or negative sentiment, or news articles by topic.
Text understanding, on the other hand, aims to go beyond classification and identify the meaning of the text by extracting relevant information, such as named entities, dates, and locations. This can be useful for information extraction, question answering, and chatbots.
Both text classification and understanding rely heavily on machine learning algorithms that learn from labeled data to make predictions on new, unlabeled data.
Sentiment analysis
This next NLP application involves identifying and extracting opinions, emotions, and attitudes expressed in text data and is used to automatically classify text as positive, negative, or neutral or to assign a sentiment score to a piece of text.
Sentiment analysis can be applied to various data types, such as social media posts, customer reviews, and news articles, to understand the public perception of a product, service, or brand.
Sentiment analysis can also be used in customer service to automatically identify negative feedback and respond to it promptly. Machine learning algorithms, such as Naive Bayes, Support Vector Machines, and neural networks, are commonly used for sentiment analysis.
Machine translation
Machine translation is an NLP application that automatically translates text from one language to another. It enables communication and understanding across different languages and cultures, facilitating international business, diplomacy, and education.
Machine translation can be rule-based, where translations are generated using a set of predefined rules, or statistical, where translations are generated based on statistical patterns in a large corpus of bilingual texts. Neural machine translation is a more recent approach that uses deep learning neural networks to generate more accurate translations.
However, despite recent advances in machine translation, it still needs to work on dealing with idiomatic expressions, ambiguous words, and syntactic differences between languages.
Chatbots and dialogue systems
Finally, chatbots and dialogue systems involve creating virtual agents that can interact with humans in natural language. Chatbots are typically used for simple, scripted interactions, such as customer service inquiries or scheduling appointments.
Dialogue systems, on the other hand, can engage in more complex and open-ended conversations, such as virtual assistants like Siri and Alexa. These systems use techniques such as natural language generation, sentiment analysis, and intent recognition to understand user queries and generate appropriate responses.
Chatbots and dialogue systems have the potential to drastically improve customer service and automate repetitive tasks, but they also face challenges such as maintaining conversational flow, handling out-of-scope requests, and ensuring data privacy and security.
Benefits of NLP
NLP has numerous benefits in various domains. For example, in business, NLP can be used for sentiment analysis to understand customer feedback and improve product offerings.
It is effective for medical record analysis, clinical decision-making, and drug discovery in healthcare, whereas in education, NLP can be used for intelligent tutoring systems, automated grading, and personalized learning.
In social media, for instance, NLP can be used for trend analysis, spam detection, and user profiling.
Overall, here are the most significant advantages of using NLP in your business:
- Improved customer experience by giving you the means to better understand customer feedback and improve your products and services accordingly;
- Streamlined workflows that you can achieve by categorizing and extracting critical information from large volumes of data, such as emails or social media posts, and automate routine tasks;
- Enhanced decision-making - the information extraction can help you get key insights and trends from data and make better decisions;
- Increased efficiency - NLP’s text generation application can help automatically generate reports, summaries, or responses to customer inquiries;
- Cost savings - by automating routine tasks and streamlining workflows, NLP can help you reduce labor costs and improve operational efficiency.
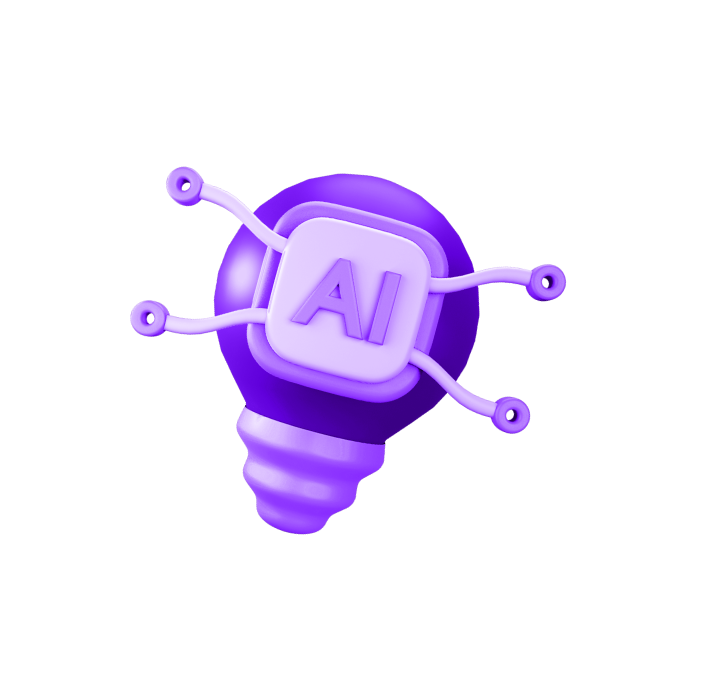
Future directions for Natural Language Processing applications
As NLP continues to advance, the future trends for its applications are becoming increasingly exciting and diverse. Here are two ways in which it’s going to go next.
Improving efficiency and accuracy
Improving efficiency and accuracy is a crucial future direction for NLP applications. This means developing more sophisticated algorithms and models that can process and analyze text more quickly and accurately, as well as improving the quality and quantity of training data.
One approach to improving efficiency is using pre-trained language models, which can be fine-tuned for specific tasks and domains.
Another method is to incorporate domain-specific knowledge and context into NLP models, enabling them to perform better on domain-specific tasks.
Incorporating multimodal input, such as speech and images, can also improve the accuracy and robustness of NLP models.
By improving efficiency and accuracy, NLP can become even more valuable in its many applications, from healthcare to education to business.
Deeper understanding of language
A deeper understanding of language is the second-most vital direction for NLP applications. It involves creating models that can understand language in a more nuanced and human-like way, considering factors such as context, emotion, and sarcasm.
One approach to achieving this is by developing neural language models that can learn from large amounts of data and represent language in a more abstract way.
Another approach is to incorporate commonsense knowledge into NLP models, enabling them to reason and make inferences about language in a more human-like way.
By achieving a deeper understanding of language, NLP models can become more versatile and effective in their many applications, from conversational agents to content analysis to machine translation.
Conclusion
In conclusion, Natural Language Processing has come a long way since its inception, and it continues to revolutionize how we interact with language.
From automatic summarization and sentiment analysis to machine translation and chatbots, we’ve learned that NLP has numerous practical applications that currently benefit businesses and individuals alike.
We know that moving forward, the future of NLP lies in improving efficiency and accuracy and achieving a deeper understanding of language, enabling even more versatile and effective applications.
As AI development processes continue to evolve and AI design companies keep pushing the boundaries, we can expect to see many exciting advancements in NLP technology in the years to come.
Stay tuned for more AI-related guides, and don’t hesitate to contact our team if you have any questions or projects you wish to bring to this world.